Hrushikesh Vazurkar
Highly motivated and adaptable data professional with a proven ability to build strong relationships through effective communication. Well-versed in agile methodology, software development, big data and machine learning.
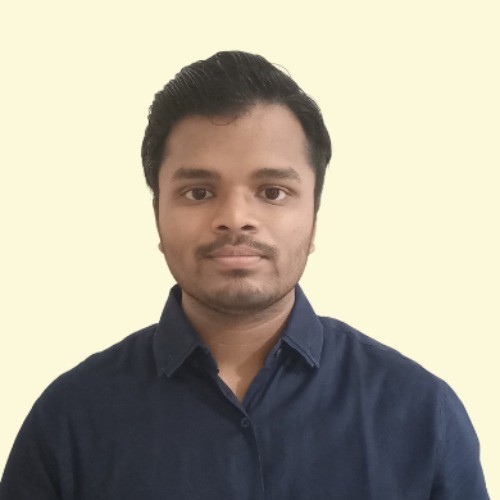
Highly motivated and adaptable data professional with a proven ability to build strong relationships through effective communication. Well-versed in agile methodology, software development, big data and machine learning.
Beyond my professional life, I'm an adventurous soul who believes in continuous learning and personal growth. When I'm not crafting elegant code solutions, you'll find me exploring new horizons and pursuing various interests.
I'm passionate about technology and its potential to make the world a better place. I believe in creating solutions that not only solve problems but also bring joy to users.
This project focused on analyzing publicly available insurance data, including Payment Protection Insurance (PPI), from the Financial Ombudsman Service (FOS). The work began with downloading FOS complaint data (PDF files) via API, processing it, and creating a structured dataset. Key achievements include uncovering temporal and insurance-specific patterns of customer complaints through exploratory data analysis (EDA). Additionally, I developed a predictive model using Distil-BERT with down-sampling techniques to replicate the FOS decisions for customer complaints, achieving an accuracy of 84%. The project culminated in a detailed report, highlighting complaint spikes over time and proposing strategies for scrutinizing insurance products based on both the likelihood of complaints being upheld and model predictions. This end-to-end workflow demonstrated expertise in data processing, advanced machine learning, and strategic analysis for actionable insights.
...Read moreThis project involved developing an unsupervised model to identify risky transactions from an hourly time-series dataset of employee spending for Lloyd’s Bank. The process began with exploratory data analysis (EDA) and time-series diagnostic tests to validate attributes such as inter-employee correlation and autocorrelation, aiding in feature engineering and model selection. Using the Isolation Forest algorithm, the model achieved optimal results, with F1 scores of 0.94 for identifying non-risky events and 0.23 for risky transactions. This project demonstrated strong skills in unsupervised learning, time-series analysis, and anomaly detection to provide actionable insights for risk management.
...Read moreI participated in a hackathon by a startup called Space Intelligence. During the course of 1-week, we performed analysis on geospatial data (GLanCE dataset and Landsat-8) for Bolivia to quantify the drivers of deforestation and influence policy change regarding potential land prime for reforestation.
...Read more